Industry Focus: Healthcare
Nurse Scheduling, Mobile Clinicians, and Predictive Analytics
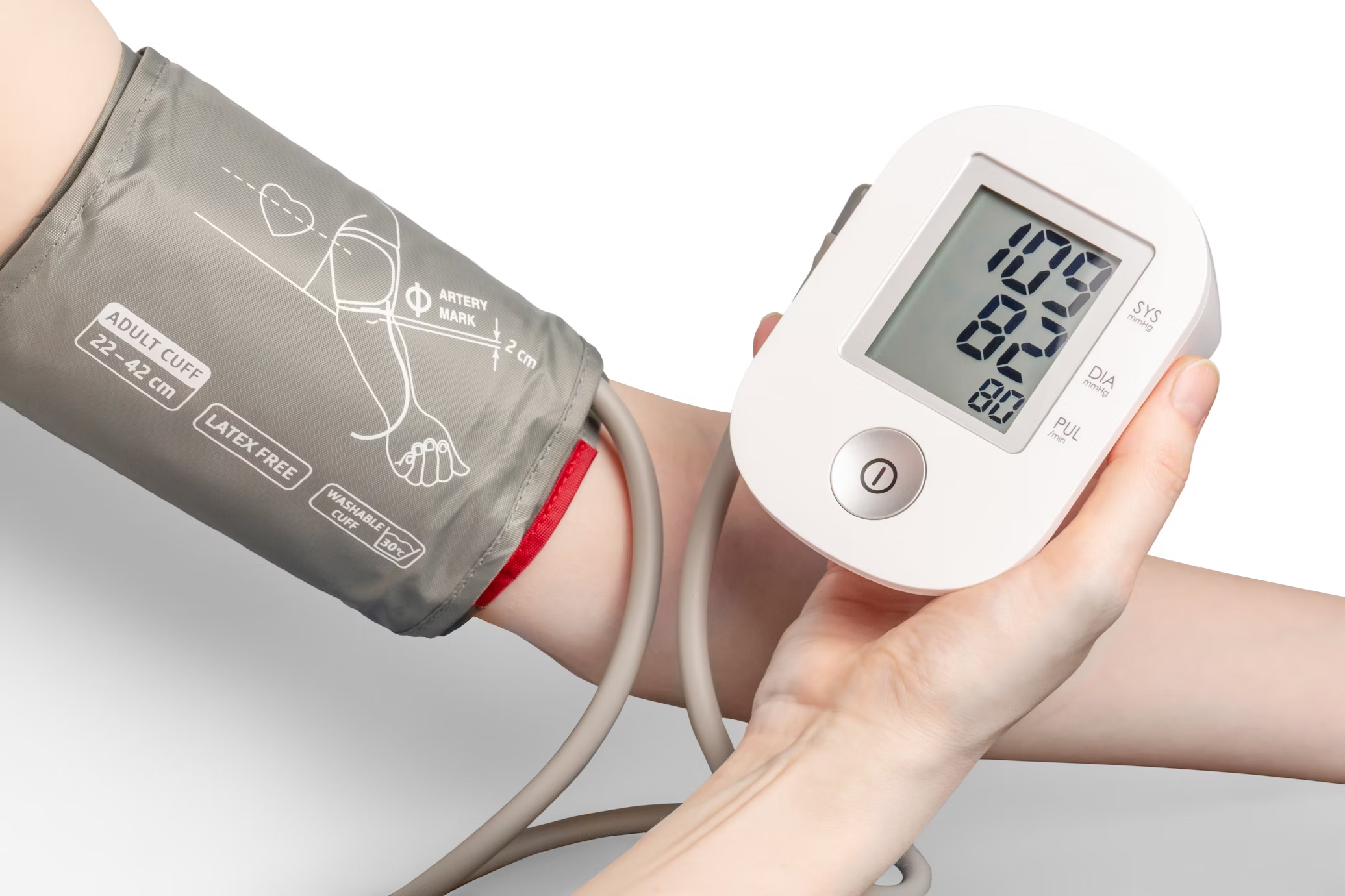
At BrightBean Labs, we specialize in creating state-of-the-art, custom software solutions for the healthcare industry. Our expertise lies in developing innovative applications that optimize operations, improve patient care, and drive significant cost savings. Below are detailed examples of our recent projects, highlighting our ability to address complex challenges and deliver tailored solutions that meet the unique needs of healthcare providers.
Project A: Nurse Scheduling Tool
Challenge: Healthcare organizations often face challenges in efficiently scheduling nurses across various departments, taking into account factors such as shift preferences, skill sets, and regulatory requirements. A hospital client struggled with manual scheduling processes that led to frequent errors, staffing shortages, and nurse dissatisfaction. Additionally, the system lacked flexibility, making it difficult to accommodate last-minute changes and emergency staffing needs.
Solution: We developed a custom nurse scheduling tool that automates the scheduling process while considering a variety of factors, including nurse availability, preferred shifts, skill levels, and compliance with labor laws. The tool uses advanced algorithms to generate optimal schedules that meet the needs of both the hospital and its staff. Furthermore, the tool includes features for real-time adjustments, allowing managers to quickly reassign shifts in case of unexpected absences or emergencies.
Outcome: The implementation of the nurse scheduling tool led to a 40% reduction in scheduling errors and a significant improvement in staff satisfaction. Nurses reported greater work-life balance, while the facility experienced a decrease in overtime costs and an increase in overall staffing efficiency.
Project B: Mobile Clinicians and the Traveling Salesman Problem
Challenge: An organization needed to optimize the routes of their clinicians who visit multiple patients each day. The challenge was akin to the Traveling Salesman Problem, where the goal is to determine the most efficient route that minimizes travel time and distance while ensuring that all patient visits are completed within specified time windows. The agency faced issues with inefficient routing, leading to increased travel costs, missed appointments, and dissatisfied patients.
Solution: We developed a custom routing algorithm tailored to the needs of clinicians, taking into account factors such as patient locations, visit duration, credentials, and time windows. The solution integrates with the organization’s existing patient management system and utilizes real-time traffic data to adjust routes dynamically. Additionally, the tool includes a user-friendly interface that allows aides to view and manage their schedules on mobile devices.
Outcome: The implementation of the routing solution resulted in a 25% reduction in travel time and a 20% decrease in fuel costs. Staff were able to visit more patients within their shifts, leading to improved patient satisfaction and better overall care. The entity also saw a reduction in missed appointments and improved operational efficiency.
Project C: Machine Learning and Disease Progression Prediction
Challenge: A healthcare provider aimed to leverage data to better predict the progression of chronic diseases among their patient population. The challenge was to develop a model that could accurately identify patients at risk of rapid disease progression, enabling early intervention and personalized treatment plans. Existing methods were insufficient, leading to delayed treatments and suboptimal patient outcomes.
Solution: We designed a machine learning model using Random Forests to analyze patient data and predict disease progression. The model was trained on a large dataset that included patient demographics, medical history, lab results, and treatment records. By identifying key predictors of disease progression, the model was able to classify patients into different risk categories. The solution also includes a dashboard for healthcare providers to monitor patient risk levels and make informed decisions about interventions.
Outcome: The machine learning model improved the accuracy of disease progression predictions by 35%, enabling healthcare providers to intervene earlier and tailor treatments to individual patients' needs. This led to better patient outcomes, including slower disease progression and reduced hospitalizations. The healthcare provider also benefited from more efficient resource allocation and improved patient management.